Last Wednesday, May 22nd, a breakfast was held that brought together representatives from Banco Credicoop, Federación Patronal, IMS Corporate, Banco Galicia and Sura Seguros to address the topic “AI: the biases that hide our data.” The talk was moderated by Marcelo Rinesi (data scientist) and Joaquín Díaz Vélez (business developer and AI department leader at Flux IT), and began with the analysis of how to implement AI in a company.
Rinesi started the meeting highlighting three ideas or ways to understand Artificial Intelligence at the business level:
“One way to see AI today is as a compiler, so anything that has code, you can look and think ‘I can use AI for this.’ Another way to understand AI is as information processing (the cognitive part) today known as Data Science.”
Regarding this second way of thinking about this technology, he added that “part of the evolution of AI is to think about what happens if each piece of a process or code can determine, by taking A and B, what will happen with C,” and he argued that, in practice, this logic is applied in micro-decisions in a massive way.
Rinesi explained that a third way of understanding AI is to think of artificial humans: chatbots, autonomous vehicles, etc.
“This is the meaning that most affects the imagination and advertising, and the application that generates the least return. It is a niche that restricts cases of possible use, and generates a lot of ethical issues that, to a certain extent, are either very early or interesting but irrelevant on a day-to-day basis.”
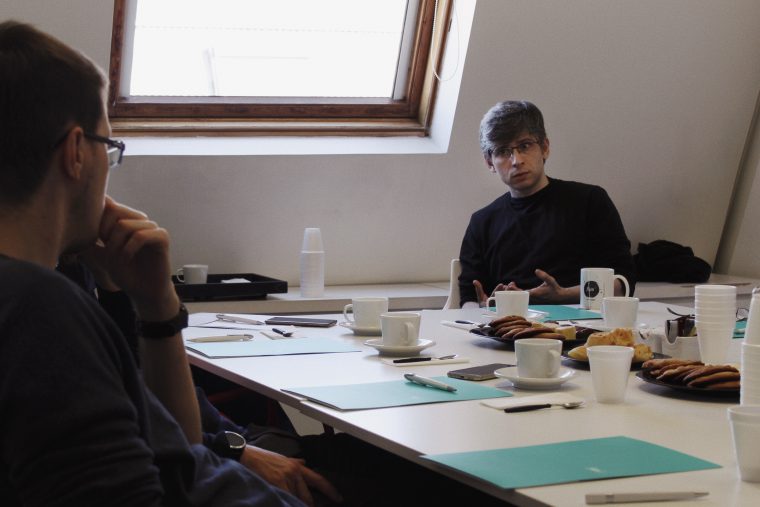
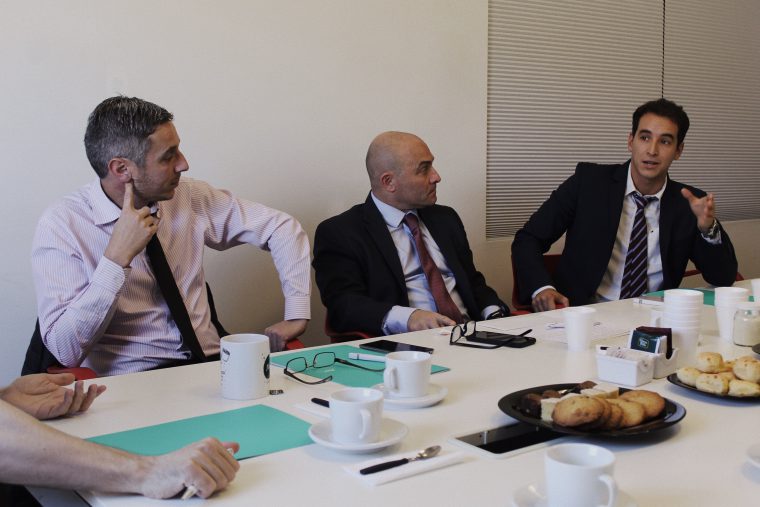
Another trigger presented by Díaz Vélez during the event was how we think about ethics when implementing AI solutions.
In relation to this issue, Rinesi argued that the privacy and ethics crises that we are seeing in AI is not due to new problems but to new actors that access the information: “The ethical problems that Facebook has with AI are not different from those that banks have today: How do I use the information? How do I keep it private? How do I prevent my systems from being used to do illegal or immoral things?”
He also assured that these issues are put into discussion because it is an unproven technology, especially in some industries: “the ethical problem of AI in banking and finance is not overuse but rather lack of use.”
During the meeting, attendees also shared their experiences applying AI to different business solutions, in dialogue with the moderators.
AI for achieving more efficient processes
“The heavy use of AI in business issues is generally not to replace a human but to replace and optimize processes.” Readdressing the initial trigger, Rinesi advanced to a crucial issue for the advance of AI in business and explained that already established companies do not need publicity, but rather efficiency of processes.
“The main problem with the use of AI in companies is not technical: technology is there, and it is light years from what is used. It is not a matter of innovation or of money. It is not even a matter of data. It is a cultural issue. The step of not using AI to using it, not only externally but internally, is a cultural transition that now generates an obstacle: by being transformative, it is traumatic; by being traumatic, not many people use it; and those who do not are falling behind.”
Referring to the financial and insurance industry, he argued that the risk is not in ethical matters, but in falling into the trap of applying this technology in a way that imitates other industries that have different needs and problems.
“The fundamental organizational skill in the 21st century (and differential in the productivity of a company) is how willing I am to change my organizational structure and my processes to empower computers. The companies that will be ahead are those that find ways to negotiate these internal issues and build things that are useful, that generate higher ROI.”
The technologist also highlighted the integration of decisions on system integration, and explained that the difference maker is not having more data, but being encouraged to try: “the power of error is greatly underestimated. You tend to see AI as something that gives certainty. AI does not prevent risk, but rather monetizes it. “
“AI does not prevent risk, but rather monetizes it.”
Marcelo Rinesi, data scientist
Finally, he emphasized the importance of defining a scope to start applying AI, and suggested starting by detecting the value that a specific department or area can generate, and then thinking at a company scale: “I recommend you think about what you can exploit that generates value and internal visibility, and then seeing what data you need (and not vice versa), so that the snowball begins to grow.”
The meeting concluded with a time for questions and consultations with the moderators.